Unlocking the Power of Data Annotation Tools and Platforms
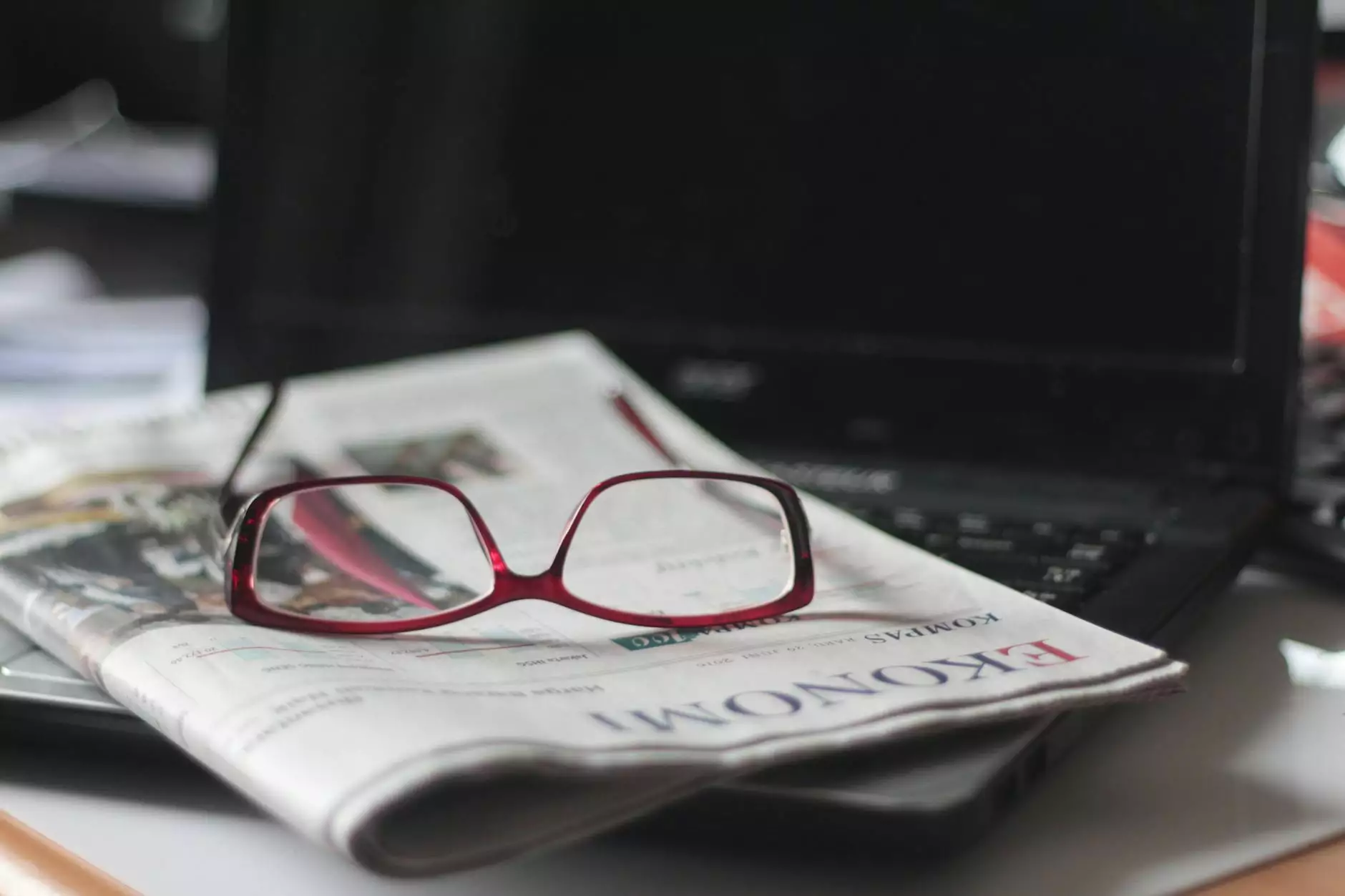
In today's fast-paced digital landscape, the importance of quality data cannot be overstated. The rise of artificial intelligence (AI) and machine learning (ML) has driven the demand for robust data annotation tools and platforms. In this comprehensive guide, we'll dive deep into the essentials of data annotation, explore various tools and platforms including KeyLabs.ai, and highlight the strategic advantages they offer.
What is Data Annotation?
Data annotation refers to the process of labeling or tagging data to make it usable for training machine learning models. This could involve classifying images, transcribing audio, or identifying key features in text. The quality of the annotated data directly impacts the performance of AI models, making efficient annotation tools critical for success.
The Necessity of Data Annotation
Machine learning algorithms learn from data, and annotated data is integral to that learning process. Here are some key reasons why data annotation is essential:
- Improved Accuracy: Well-annotated data enhances model performance and reduces error rates.
- Robustness: Diverse and accurately labeled data enables models to generalize better across various inputs.
- Faster Development: Automated and efficient annotation processes speed up the development of AI applications.
Types of Data Annotation
Data annotation comes in various forms, each serving different purposes:
1. Image Annotation
Image annotation involves labeling images for tasks such as object detection, segmentation, and classification. This is particularly vital for self-driving cars and facial recognition systems.
2. Text Annotation
Text annotation encompasses labeling aspects of text data, including named entity recognition (NER), part-of-speech tagging, and sentiment analysis. This is crucial for natural language processing (NLP) applications.
3. Video Annotation
Video annotation combines image and audio annotation to label moving images over time, making it essential for tasks in surveillance, behavior analysis, and more.
4. Audio Annotation
Audio annotation entails transcribing spoken words, marking phonetics or environmental sounds. This is key for voice recognition systems and automated transcription services.
The Advantages of Using Data Annotation Tools
Using dedicated tools for data annotation can transform the way businesses handle data. Here are some major advantages:
- Efficiency: Tools streamline the annotation process, allowing for faster completion of projects and better resource management.
- Scalability: With powerful platforms, organizations can scale their annotation efforts to meet the growing demands of their projects.
- Collaboration: Many tools enable multiple team members to collaborate efficiently, improving the quality of annotation through diverse inputs.
- Integration: Seamless integration with existing workflows and AI frameworks enhances operational fluidity.
Overview of KeyLabs.ai
KeyLabs.ai specializes in advanced data annotation solutions tailored for AI and machine learning needs. Their platform offers a variety of features designed to maximize the quality and efficiency of data handling. With an emphasis on user-friendly interfaces and cutting-edge technology, KeyLabs.ai stands out in the competitive landscape of data annotation tools.
Key Features of KeyLabs.ai
The following features highlight why KeyLabs.ai is a game-changer in the data annotation arena:
- Automated Annotation: Harnessing machine learning capabilities to automate time-consuming tasks.
- Customizable Workflows: Tailoring the annotation process to fit unique project requirements.
- Quality Assurance: Implementing rigorous validation processes to ensure high-quality annotated data.
- Flexible Data Formats: Accepting a wide array of input formats, facilitating versatility in projects.
- Collaboration Tools: Enabling teams to work together efficiently, providing real-time feedback and improvements.
Choosing the Right Data Annotation Platform
With numerous data annotation tools and platforms available, choosing the right one can be challenging. Here are key considerations:
1. Quality of Annotation
The most important aspect of any data annotation tool is the quality of the annotations it produces. Look for platforms with proven methodologies and quality assurance processes.
2. User Experience
The interface should be intuitive and easy to navigate, reducing the learning curve for new users and increasing productivity.
3. Integration Capabilities
Ensure that the platform can seamlessly integrate with your existing data pipelines and machine learning frameworks.
4. Scalability
As your projects grow, is the platform capable of scaling alongside? Choose a tool that can adapt to your changing needs.
5. Cost
Consider your budget and evaluate the return on investment for choosing a particular platform. Free trials are often useful to assess functionality without financial commitment.
Tips for Effective Data Annotation
Maximizing the effectiveness of your data annotation efforts requires strategic planning and execution. Here are some tips to guide you:
- Define Clear Guidelines: Establishing standard operating procedures for annotation ensures consistency and quality.
- Invest in Training: Training annotators thoroughly can significantly improve the outcomes of your annotation efforts.
- Use Technology Wisely: Leverage automated tools when appropriate to free up human resources for more complex tasks.
- Conduct Regular Reviews: Frequent assessments of your annotated datasets can help identify problems and areas for improvement.
- Engage in Continuous Learning: Stay updated with the latest trends and technologies in data annotation and the broader AI landscape.
Future Trends in Data Annotation
The field of data annotation is rapidly evolving, with emerging trends that are shaping its future:
1. Increased Automation
As AI improves, automation will play an increasingly significant role in data annotation, minimizing human intervention and expediting the process.
2. Real-time Annotation
Future tools may offer capabilities for real-time annotation, enabling immediate feedback and adjustments during the data collection process.
3. Enhanced Collaboration Tools
As remote work becomes more common, the need for robust collaborative features in data annotation tools will grow.
4. Focus on Quality over Quantity
Organizations will prioritize quality, seeking tools that ensure high-standard annotations rather than simply high-volume outputs.
5. Ethical Considerations
With increased scrutiny on AI outputs, ethical data annotation practices will become even more critical, ensuring fairness, accuracy, and transparency.
Conclusion
In conclusion, data annotation is a foundational element of any successful AI or machine learning project. By leveraging advanced tools like KeyLabs.ai, businesses can optimize their data handling processes, improve accuracy, and ultimately achieve their strategic goals. The focus on quality, efficiency, and future-readiness will position organizations for success in an increasingly data-driven world.
As you embark on your data journey, remember that informed decision-making regarding data annotation platforms will pay dividends in your AI projects.
https://keylabs.ai/